

Two of the most established methods are the thermal signal reconstruction (TSR) and the pulsed phase thermography (PPT) algorithms. In this regard, different methods, based on one-dimensional models, are used in the literature to estimate the depth and size of defects. Subsurface defects can be detected by the pulsed thermography (PT) technique analysing the raw thermal data with the application of different post-processing algorithms. Experiments on a panel painting illustrate that the proposed method promotes the accuracy and efficiency of thermographic detection of defects, compared with the popular principal component thermography (PCT) method. Additionally, the parameter selection is emphasised and addressed.
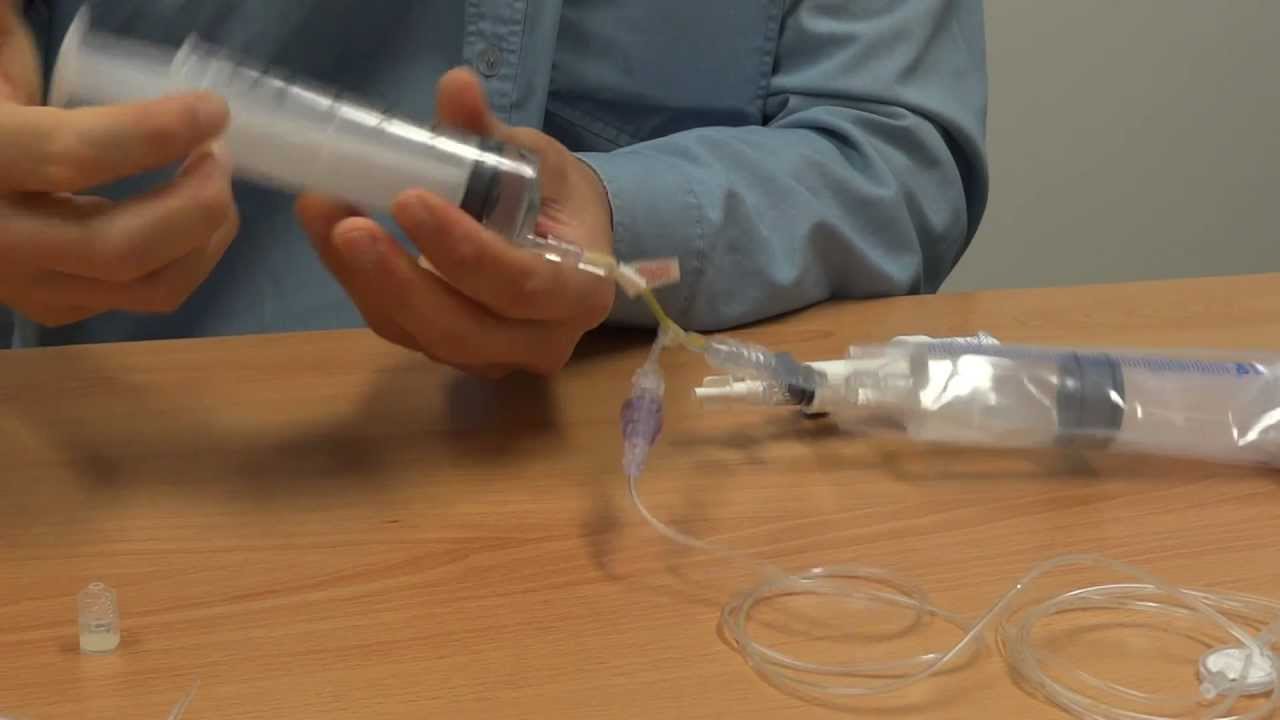
Employing the FCM image segmentation algorithm on factor loading images reduces the interference of background on human visual detection. Consequently, the defect information is extracted while large signal-to-noise ratios are obtained. By means of factor rotation, factor analysis minimises the complexity of factor loadings and makes the results more interpretable. This work proposes to use a factor analysis thermography (FAT) method that automatically extracts defect features from thermograms via exploratory factor analysis, in tandem with a fuzzy c-means (FCM) clustering algorithm to segment the defects and background. In many applications, thermographic data processing is necessary to extract defect features from a large number of thermal images. The result of the proposed method suggests that the embedding can help preserve important thermal patterns, which significantly aid CBE and early detection of breast cancer.Īctive infrared thermography is an important non-destructive testing method used for revealing defect structures in materials. The results of the proposed method combining clinical information, and demographics yield 78.9% (75.7%, 85.9%), while the convex-NMF alone gave 76.9% (73.7%, 86.1%). A comparison analysis on different embedding methods and factorization approaches is also provided. This low dimensional (LD) representation of a thermal sequence was then used to extract thermomics and train tuned hyperparameters random forest model for early breast cancer diagnosis. This study presents the applications of convex factor analysis combined with the bell-curve membership function embedding approach to tackle this task and generate one image to represent the entire sequence. Finding a single predominant infrared image capturing the prevalent patterns of changes remains a challenging task in the field. These methods are often used to summarize thermographic sequences and simultaneously highlight predominant thermal patterns. There are many matrix factorization methods developed for computational thermography that can be used to extract thermal variations across the acquisition time. Thermographic imaging has proven to be effective for the early detection of breast cancer and with clinical breast examination (CBE). The results conclusively demonstrate the practical efficacy of the approach, and confirm the attractive properties outlined in relation to the numerical tests. An AS4/3501 composite laminate sample containing an assortment of teflon inserts is subject to active thermographic inspection and the response data analysed using the proposed approach.
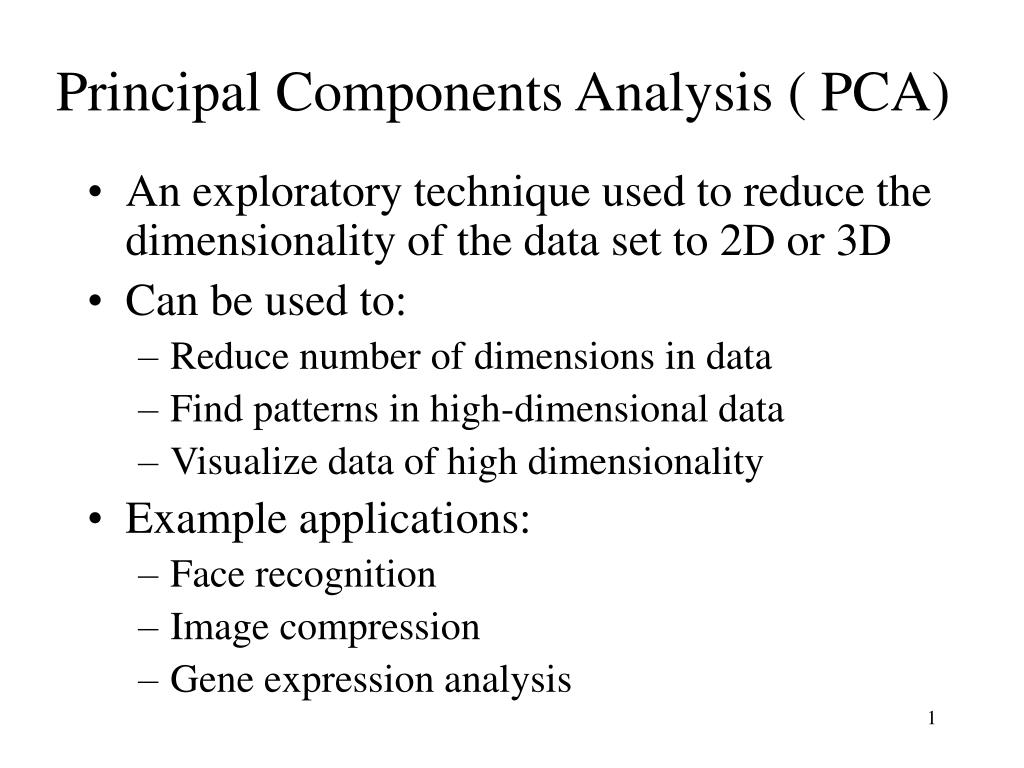
Finally, application to experimental data is considered. This framework is shown to provide flaw-depth estimates with good levels of precision and robustness. A simple analytical expression is then derived that relates a characteristic time furnished by the decomposition to the flaw depth, providing a basis for flaw depth estimation. Trials on synthetically generated data show evidence of excellent noise-rejection qualities which manifest high levels of flaw contrast relative to that present in the unprocessed data. The technique is based on a singular value decomposition of the measured response to pulsed thermal excitation as applied in the course of a normal flash thermographic inspection. This study reports on the application of principal component thermography to the nondestructive inspection of composite structures.
